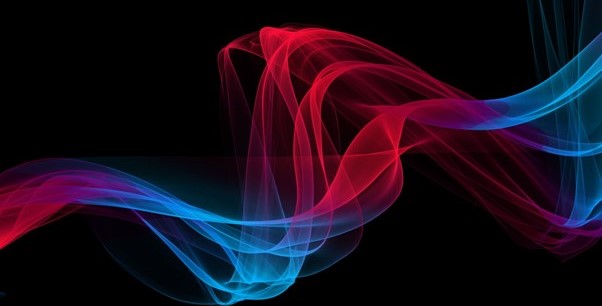
P-Hacking or data dredging is the practice of analyzing data until you find the results needed to back up your hypothesis. However, in many cases this practice is unintentional and researchers follow the P-hacking route without fully realizing they are doing it.
The scientific method is built on formulating a hypothesis, designing the study, running the study, analyzing the data and then publishing the study (1). Through the strict adherence to these steps in a transparent and clear manner, what is published will provide evidence for the initial hypothesis or not. In most cases, the published results are positive and this is where the issue of P-hacking comes in. During the analysis stage, researchers can identify positive results when there isn’t any. As Munafo, et al. states, whilst scientists should be open to new and important insights they need to simultaneously avoid being led astray by the tendency to see structure in randomness (2).
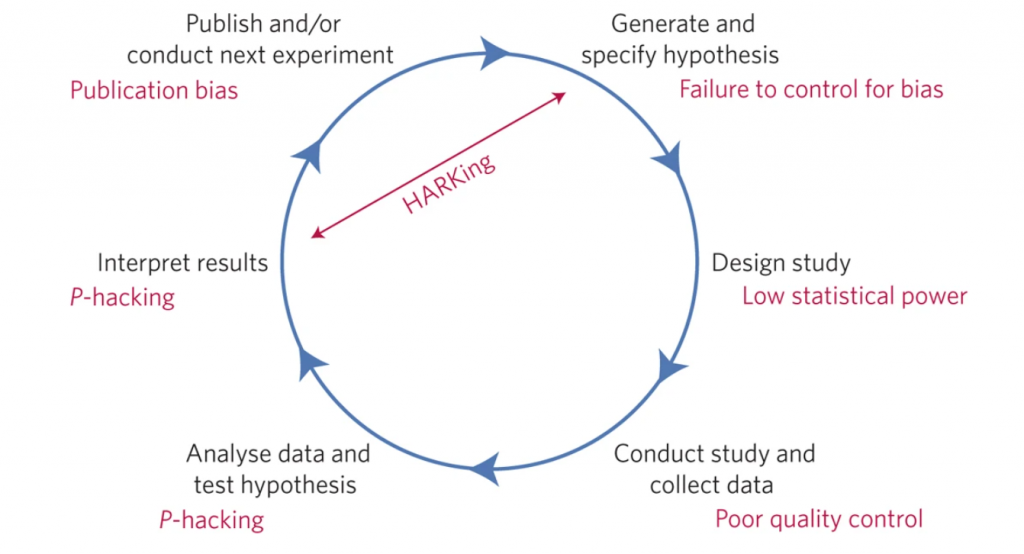
P-hacking can derive from apophenia, confirmation bias and hindsight bias. Apophenia is when people pick up patterns in random data, they are looking for a significant result and will keep looking until it is ‘discovered’. The inbuilt basis is amplified by the makeup of data analysis, there are a multitude of ways in analyzing the same data, making it more likely to identify false positives. Hypotheses may emerge that fit the data and are then reported without indication or recognition that you are hypothezing after the results (3)
How then can we prevent P-hacking?
To avoid cognitive bias you can introduce a blinding element into the process, such as between the data analysis and key parts of the data. Improved statistical training and being able to replicate the result from the same sample size. Research reproducibility is key to ensuring that identifying a significant result can be reproduced with a clear and transparent methodology and study design. Preregistration was introduced to tackle publication bias, ensuring that papers are published regardless of the final outcome of the study, it can also be used against P-hacking by preventing the outcome being switched during data analysis, as the proposed study has already been registered and needs to be followed all the way through. There is a wider issue around research culture which sways towards competitiveness rather than collaboration. Researchers are under pressure to publish papers and with most journals, publication is tied to positive and not negative or null results. Until there is less push and pull to publish papers that confirm a novel hypothesis rather than through robust scientific method, then p-hacking will be difficult to avoid.
References
- Bezak, et al. The Open Science Training Book. TIB, Hannover Germany (2018) https://doi.org/10.5281/zenodo.1212496
- Munafò, M., Nosek, B., Bishop, D. et al. A manifesto for reproducible science. Nat Hum Behav 1, 0021 (2017). https://doi.org/10.1038/s41562-016-0021
- Kerr, N. L., HARKing: hypothesizing after the results are known. Pers. Soc. Psychol. Rev. 2, 196–217 (1998).